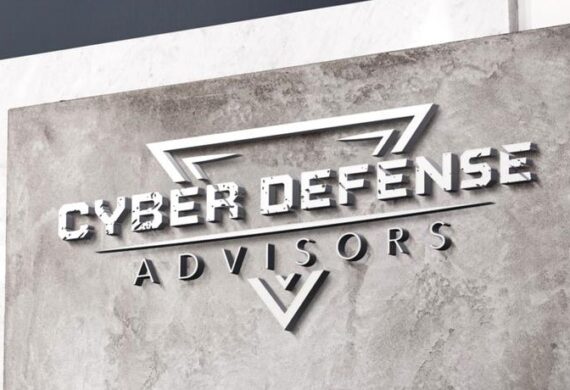
HIPAA Compliance in AI-driven Predictive Health Analysis
In an era where data drives innovation across all sectors, the healthcare industry is no exception. The rapid advancement of artificial intelligence (AI) has enabled healthcare professionals to harness vast amounts of patient data for predictive health analysis, diagnosis, and treatment planning. While these AI-driven solutions hold immense promise for improving patient care, they also raise critical concerns about patient privacy and data security. This is where HIPAA (Health Insurance Portability and Accountability Act) compliance becomes paramount.
Understanding HIPAA: A Brief Overview
HIPAA, enacted in 1996, is a federal law in the United States designed to protect sensitive patient information, also known as Protected Health Information (PHI). Its primary objectives are to ensure the confidentiality, integrity, and availability of patient data while facilitating the secure exchange of health information. HIPAA comprises several rules and provisions, but two primary regulations are particularly relevant to AI-driven predictive health analysis:
- HIPAA Privacy Rule: This rule sets standards for the protection of PHI and defines who can access and share this information. It establishes the patient’s right to control their health information and limits the use and disclosure of PHI without patient consent.
- HIPAA Security Rule: This rule outlines safeguards for protecting electronic PHI (ePHI) and requires entities subject to HIPAA to implement administrative, physical, and technical measures to ensure the confidentiality, integrity, and availability of ePHI.
Now, let’s delve into the complexities of integrating AI-driven predictive health analysis into healthcare systems while maintaining HIPAA compliance.
AI in Healthcare: Transforming Predictive Health Analysis
AI technologies, including machine learning and deep learning algorithms, have shown remarkable capabilities in analyzing vast datasets to predict health outcomes, identify potential diseases, and optimize treatment plans. Here’s how AI is transforming predictive health analysis:
- Early Disease Detection: AI algorithms can sift through patient data, including electronic health records (EHRs), medical images, and genomic information, to detect patterns indicative of early-stage diseases. This early detection can lead to more effective interventions and improved patient outcomes.
- Personalized Treatment Plans: AI can analyze patient-specific data to develop personalized treatment plans, considering factors like genetics, lifestyle, and previous medical history. This tailored approach can enhance treatment effectiveness and minimize adverse effects.
- Resource Allocation: Predictive analytics powered by AI can assist healthcare providers in optimizing resource allocation, such as hospital beds, staff, and medical equipment, based on predicted patient admissions and disease trends.
- Drug Discovery: AI-driven algorithms can accelerate drug discovery processes by identifying potential drug candidates and predicting their effectiveness, significantly reducing the time and cost involved in drug development.
- Remote Monitoring: AI-powered wearables and remote monitoring devices enable continuous tracking of patients’ vital signs and health metrics, providing real-time data to healthcare providers for timely interventions.
While AI holds the promise of revolutionizing healthcare, its integration into the industry must adhere to HIPAA regulations to safeguard patient privacy and data security.
HIPAA Compliance in AI-driven Predictive Health Analysis
Ensuring HIPAA compliance in the context of AI-driven predictive health analysis is a complex endeavor that requires a multi-faceted approach. Here are some key considerations:
- Data Encryption: Encrypting patient data at rest and in transit is a fundamental requirement under the HIPAA Security Rule. AI systems must utilize robust encryption methods to protect ePHI from unauthorized access.
- Access Controls: Implement strict access controls to limit who can access patient data. AI algorithms should only be accessible to authorized personnel, and proper authentication mechanisms should be in place.
- Data Minimization: Collect and use only the minimum necessary patient data for AI analysis. Limiting the scope of data collection helps reduce the risk of unauthorized exposure of sensitive information.
- Audit Trails: Maintain detailed audit trails of all data accesses and modifications. This is essential for tracking any unauthorized or suspicious activities and ensuring accountability.
- Secure Cloud Storage: If AI-driven predictive health analysis relies on cloud computing, healthcare providers should select HIPAA-compliant cloud service providers that offer robust security measures.
- Training and Awareness: Train healthcare staff and AI developers on HIPAA compliance and data security best practices. Promoting awareness of these issues is crucial in preventing data breaches.
- Data De-identification: Consider de-identifying patient data before using it in AI models. This involves removing or transforming identifiable information to reduce the risk of patient re-identification.
- Business Associate Agreements: When working with third-party vendors, such as AI solution providers, establish Business Associate Agreements (BAAs) to ensure they also comply with HIPAA regulations.
- Regular Audits and Risk Assessments: Conduct regular security audits and risk assessments to identify vulnerabilities and take corrective actions promptly.
Challenges and Future Directions
HIPAA compliance in AI-driven predictive health analysis poses several challenges. AI models often require large datasets for training, and sharing such data while maintaining patient privacy is a complex task. Additionally, the evolving nature of AI algorithms and technologies means that compliance requirements may change over time.
Looking ahead, several trends may shape the landscape of HIPAA compliance in AI-driven healthcare:
- Federated Learning: This approach allows AI models to be trained on decentralized data sources, reducing the need for data sharing and enhancing patient privacy.
- Blockchain Technology: Blockchain’s immutable and decentralized nature can provide a secure platform for managing patient consent and data access permissions.
- AI Explainability: Ensuring that AI models are explainable and transparent can help build trust among patients and regulatory authorities.
- Interoperability Standards: Wider adoption of interoperability standards can facilitate secure data exchange between healthcare systems and AI applications.
In conclusion, AI-driven predictive health analysis has the potential to revolutionize healthcare by improving early disease detection, personalizing treatment plans, and optimizing resource allocation. However, healthcare providers and AI developers must navigate the complex landscape of HIPAA compliance to ensure patient privacy and data security. By adhering to stringent security measures, staying informed about evolving regulations, and adopting emerging technologies, the healthcare industry can harness the power of AI while safeguarding patient information and trust.
Contact Cyber Defense Advisors to learn more about our HIPAA Compliance solutions.