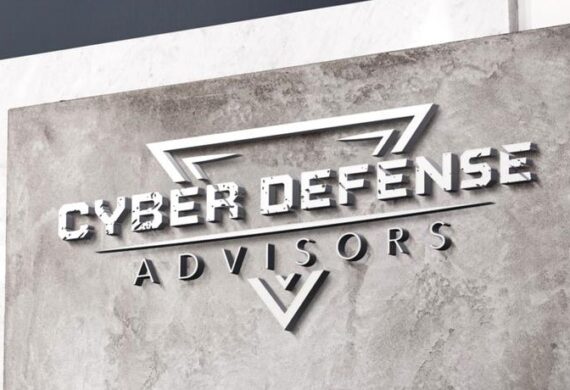
Automating Vulnerability Assessment with Machine Learning Algorithms
In an era where data breaches and cyberattacks are becoming increasingly common, the importance of robust cybersecurity measures cannot be overstated. Organizations of all sizes are constantly at risk of falling victim to malicious actors seeking to exploit vulnerabilities in their digital infrastructure. To stay ahead of these threats, businesses and security professionals are turning to advanced technologies, such as machine learning algorithms, to automate vulnerability assessment processes and enhance their cybersecurity defenses.
Understanding Vulnerability Assessment
Before delving into the role of machine learning algorithms in automating vulnerability assessment, it’s crucial to grasp what vulnerability assessment entails. Vulnerability assessment is the practice of identifying weaknesses or vulnerabilities in a computer system, network, or application. These vulnerabilities, if left unaddressed, can be exploited by cybercriminals to gain unauthorized access, steal sensitive data, or disrupt operations.
Traditionally, vulnerability assessments were conducted manually, involving the painstaking process of identifying, categorizing, and prioritizing vulnerabilities. Security experts would scan systems, analyze logs, and assess configurations to pinpoint potential weaknesses. However, with the rapid growth of digital ecosystems, this manual approach has become increasingly impractical and time-consuming.
The Role of Machine Learning in Vulnerability Assessment
Machine learning, a subset of artificial intelligence, is transforming the way organizations approach vulnerability assessment. By leveraging the power of machine learning algorithms, cybersecurity teams can automate and optimize the process of identifying and mitigating vulnerabilities. Here’s how:
- Data Analysis and Pattern Recognition: Machine learning algorithms excel at processing vast amounts of data and recognizing patterns. Security professionals can feed these algorithms with data from various sources, including system logs, network traffic, and historical attack data. The algorithms can then analyze this data to identify anomalies or suspicious activities that might indicate potential vulnerabilities.
- Predictive Analysis: Machine learning models can predict which vulnerabilities are most likely to be exploited based on historical data and emerging threat trends. By prioritizing vulnerabilities that are more likely to be targeted, organizations can allocate their resources more effectively and address high-risk vulnerabilities first.
- Real-time Monitoring: Machine learning algorithms can provide real-time monitoring of network traffic and system activity. They can detect and respond to threats as they happen, helping organizations take immediate action to mitigate vulnerabilities and protect their systems.
- Automated Vulnerability Scanning: Machine learning can automate the process of scanning systems and applications for known vulnerabilities. These algorithms can quickly identify weaknesses and provide detailed reports to security teams, enabling faster remediation.
Challenges and Considerations
While machine learning offers significant advantages in automating vulnerability assessment, it’s not without its challenges and considerations:
- Data Quality: The effectiveness of machine learning algorithms depends heavily on the quality of the data they are trained on. Inaccurate or incomplete data can lead to false positives and negatives, undermining the reliability of the assessment.
- False Positives: Machine learning models may sometimes generate false positives, flagging benign activities as potential vulnerabilities. Human oversight is essential to verify and validate the results produced by these algorithms.
- Evolving Threat Landscape: Cyber threats are constantly evolving, and machine learning models must be regularly updated to stay relevant. Security teams need to keep their algorithms up to date with the latest threat intelligence.
- Integration and Compatibility: Implementing machine learning algorithms into existing cybersecurity infrastructure can be complex. Compatibility issues and the need for integration with existing tools must be carefully managed.
Case Studies
Several organizations and security firms have already started using machine learning to automate vulnerability assessment:
- Darktrace: Darktrace’s Enterprise Immune System employs machine learning to detect and respond to cyber threats in real-time. It learns the normal behavior of an organization’s network and can identify anomalies that may signify vulnerabilities or attacks.
- Qualys: Qualys, a cybersecurity company, offers a cloud-based platform that uses machine learning to automate vulnerability management. It scans networks for vulnerabilities, prioritizes them based on risk, and provides remediation guidance.
- Tenable: Tenable’s vulnerability management solutions use machine learning to identify and prioritize vulnerabilities in an organization’s IT environment. It offers predictive analytics to help organizations proactively address potential threats.
The Future of Vulnerability Assessment
As the cyber threat landscape continues to evolve, the automation of vulnerability assessment through machine learning will become increasingly vital. With machine learning algorithms at the helm, organizations can strengthen their cybersecurity posture by identifying and addressing vulnerabilities faster and more efficiently.
Looking ahead, we can expect further advancements in machine learning technology, including enhanced predictive capabilities, improved accuracy, and better integration with existing security infrastructure. However, it’s crucial to remember that while machine learning can automate many aspects of vulnerability assessment, human expertise remains a critical component of effective cybersecurity. Human analysts are needed to interpret results, make strategic decisions, and adapt to emerging threats.
In conclusion, automating vulnerability assessment with machine learning algorithms is a significant step forward in enhancing cybersecurity. It enables organizations to stay ahead of cyber threats, identify vulnerabilities proactively, and prioritize remediation efforts. As technology continues to evolve, the synergy between human expertise and machine learning will be essential in building robust defenses against an ever-changing cyber threat landscape.
Contact Cyber Defense Advisors to learn more about our Vulnerability Assessment solutions.