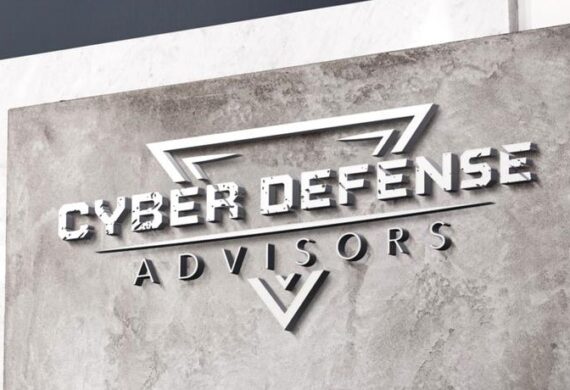
An AI Integration for Applications Checklist
Artificial Intelligence (AI) has become a transformative force across industries, revolutionizing the way businesses operate and individuals interact with technology. Integrating AI into applications has become a strategic imperative for organizations aiming to stay competitive and provide enhanced user experiences. However, the process of AI integration can be complex and fraught with challenges. To navigate this journey successfully, it is crucial to have a well-defined checklist that guides you through the process. In this article, we present an AI Integration for Applications Checklist to help you plan and execute your AI integration efforts effectively.
- Define Clear Objectives
Before embarking on an AI integration project, it’s essential to have a clear understanding of your objectives. Ask yourself what specific problems or tasks you intend to solve with AI. Are you looking to automate repetitive tasks, improve user experiences, enhance data analytics, or gain deeper insights from your data? Defining your objectives will serve as the foundation for the rest of your AI integration efforts. - Assess Data Availability and Quality
AI algorithms rely heavily on data for training and making predictions. Evaluate the availability and quality of your data sources. Ensure that you have access to a sufficient volume of relevant data. Also, consider data quality, consistency, and potential biases. Data preparation and preprocessing are often the most time-consuming aspects of AI integration, so it’s vital to start with a solid data foundation. - Choose the Right AI Technologies
Selecting the appropriate AI technologies is a critical decision. Consider the type of AI you need – whether it’s machine learning, natural language processing, computer vision, or a combination. Additionally, decide between cloud-based AI services, pre-trained models, or building custom solutions. Your choice should align with your objectives, budget, and technical expertise. - Understand Regulatory and Ethical Considerations
AI integration is not just a technical endeavor; it also involves navigating legal and ethical landscapes. Depending on your industry and geographical location, you may need to comply with specific regulations such as GDPR or HIPAA. Ensure that your AI implementation respects user privacy, handles sensitive data responsibly, and adheres to ethical guidelines. - Build or Buy AI Models
Determine whether you should build your AI models in-house or leverage pre-built solutions. Building custom models allows for greater customization and control but requires substantial expertise and resources. Conversely, buying pre-trained models or using AI services can accelerate development but may have limitations in terms of adaptability to specific use cases. - Evaluate Model Performance
Once you have chosen or built AI models, thoroughly evaluate their performance. Use appropriate metrics for your specific objectives, such as accuracy, precision, recall, or F1-score for classification tasks. For regression tasks, consider metrics like Mean Absolute Error or Root Mean Square Error. Continuously monitor and fine-tune your models to maintain optimal performance. - Ensure Scalability and Integration
Plan for scalability from the outset. Consider how the AI components will integrate into your existing applications and systems. Ensure that the AI infrastructure can handle increased workloads as your application grows. Scalability is essential to avoid performance bottlenecks and maintain a seamless user experience. - Address Security Concerns
AI integration introduces new security considerations. Protect against potential vulnerabilities, data breaches, and adversarial attacks. Implement robust authentication and authorization mechanisms to safeguard AI models and data. Regularly update and patch AI-related software to address security vulnerabilities. - Provide Adequate Training and Documentation
Both your development team and end-users should have access to training and documentation. Ensure that your developers are proficient in AI technologies and have access to resources for troubleshooting and support. For end-users, provide clear and user-friendly documentation to help them understand how to interact with AI-powered features. - Implement Monitoring and Maintenance
AI integration is not a one-time effort; it requires ongoing monitoring and maintenance. Set up monitoring systems to track model performance, detect anomalies, and trigger alerts. Establish a maintenance schedule to update models, retrain them with new data, and apply necessary improvements to adapt to changing conditions. - User Testing and Feedback
Before deploying AI-integrated applications to a broader audience, conduct thorough user testing. Gather feedback from users to identify any usability issues, performance concerns, or areas for improvement. User feedback is invaluable for refining your AI-powered features and ensuring they meet user expectations. - Compliance and Ethics
Regularly review and update your AI models and applications to ensure compliance with evolving regulations and ethical standards. Pay close attention to any changes in data privacy laws and adapt your AI integration accordingly. Ethical considerations, such as bias and fairness in AI, should also be an ongoing focus. - Documentation and Version Control
Maintain clear documentation of your AI models, data preprocessing pipelines, and integration processes. Use version control systems to keep track of changes and revisions. This documentation and version control are essential for reproducibility, collaboration, and troubleshooting. - Cost Management
AI integration comes with costs, including infrastructure, licensing, development, and maintenance expenses. Create a budget that accounts for these costs and regularly review it to ensure that you are staying within your financial constraints. Optimize resource usage to maximize cost-efficiency. - User Education
Educate your users about the AI capabilities within your applications. Provide tutorials, tooltips, or in-app guidance to help users make the most of AI-powered features. Clear communication can enhance user adoption and satisfaction. - Plan for Failure and Contingencies
Despite careful planning, AI integration projects can encounter unforeseen challenges. Develop contingency plans for potential failures or disruptions. This may include fallback mechanisms, disaster recovery strategies, or alternative AI models. - Stay Informed About AI Advancements
AI is a rapidly evolving field. Stay informed about the latest advancements, best practices, and emerging trends in AI integration. Continuous learning and adaptation are essential to keep your AI-integrated applications competitive and up-to-date.
Conclusion
Integrating AI into applications is a multifaceted process that requires careful planning and execution. By following this AI Integration for Applications Checklist, you can streamline your efforts, mitigate risks, and maximize the benefits of AI for your organization. Remember that AI integration is an ongoing journey that requires continuous monitoring, improvement, and adaptation to stay ahead in the ever-evolving landscape of artificial intelligence.
Contact Cyber Defense Advisors to learn more about our AI Integration for Applications solutions.